Members of Sublinear-time Modeling Group
Name | Affiliation | Role |
Kazuyuki Tanaka | Tohoku University | Group Leader |
Ayumi Shinohara | Tohoku University | Member |
Hidetoshi Nishimori | Tokyo Institute of Technology | Member |
Takehiro Ito | Tohoku University | Member |
Yuji Waizumi | Tohoku University | Member |
Muneki Yasuda | Yamagata University | Member |
Akiyoshi Shioura | Tokyo Institute of Technology | Member |
Shun Kataoka | Tohoku University | Member |
Akira Suzuki | Tohoku University | Member |
Kazuyuki Narisawa | Tohoku University | Member |
Masayuki Ohzeki | Kyoto University | Member |
Research Theme: Sublinear Modeling of Big Data through a Fusion Approach of Statistical Mechanics and Computational Theory
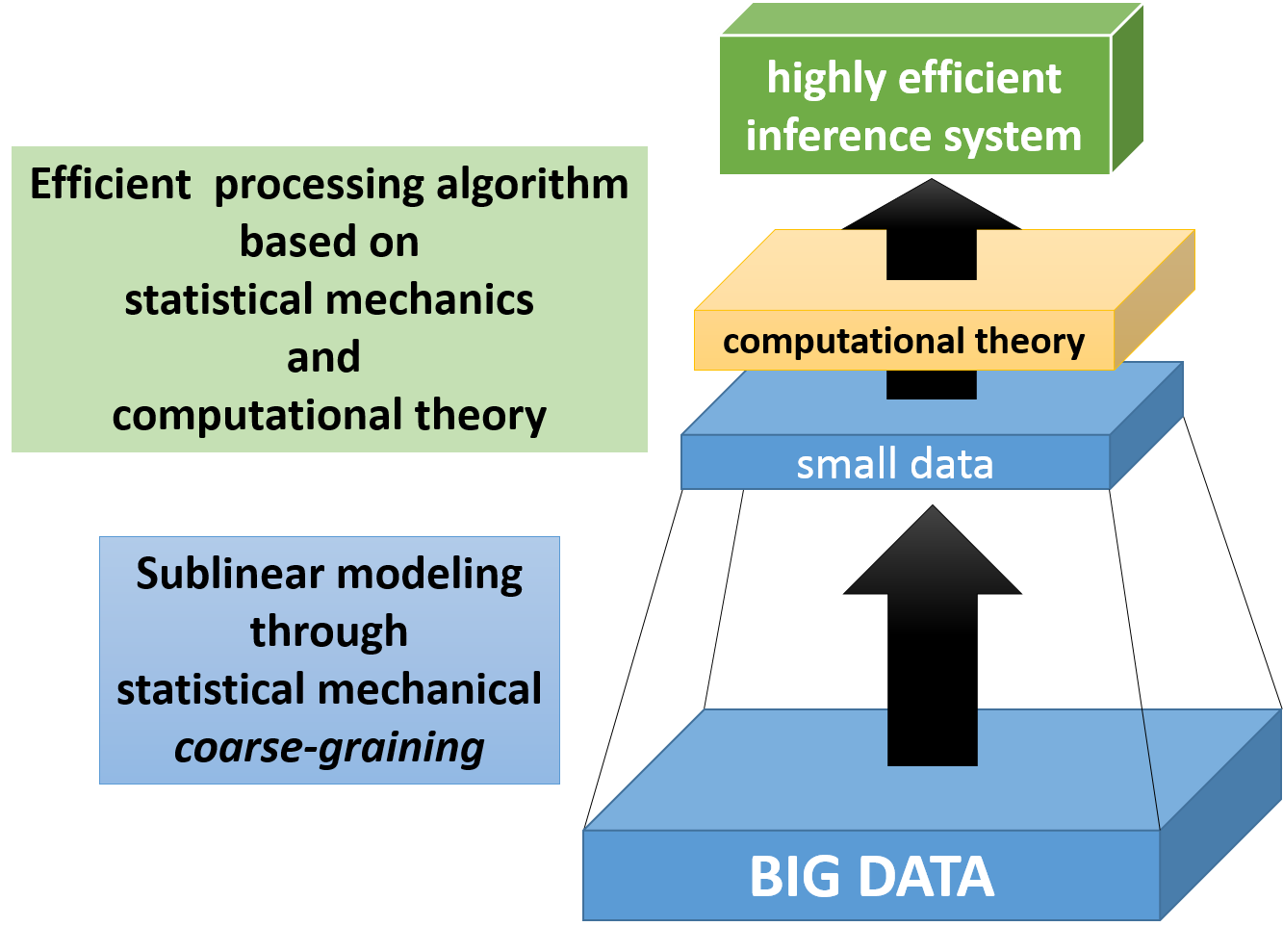
In this group, we will be proposing a new universal sublinear modeling paradigm for big data wherein we combine a statistical mechanical data coarse-graining method and a stochastic data processing theory, which is currently prominent in the field of data science.
We will also be developing a statistical prediction model that handles big data based on linear modeling as well as applications for the same, with the ultimate goal of constructing a highly efficient approximate calculation algorithm based on the proposed model.
- Big Data Sublinear Modeling through a Statistical Mechanical Coarse-Graining Approach
A sublinear approach is required to make practical use of super large-scale big data.
Therefore, in this research, we aim at constructing a robust universal theory for modeling based on data compression modeling and statistical mechanical insights into big data; that is, a statistical mechanical method of mapping large scale data onto a small scale system.
Accordingly, we have made use of methodological approaches for data coarse-graining such as mean-field theory and renormalization theory which have been developed in statistical mechanics.
- Theory of Sublinear Sparse Structure Extraction from Big Data
Identifying sparse (small number of important) correlations can help significantly reduce the volume of big data to be handled.
For example, let us assume there are sparse relationships within a given set of big data.
Accordingly, there will be no other associations between the data other than the small number of data that have a sparse relationship.
The data without associations can then be processed individually (or ignored, depending on the case), thereby enabling much faster overall data processing.
In this study, we aim to develop a method for reducing the volume of data to be handled using an approach based on regularization theory and statistical mechanics theory, which are methods within the fields of statistics and machine learning theory, to extract sparse correlations within big data.
- Big Data Clustering Theory Using a Bayesian Approach
Categorizing individual data within big data based on their characteristics (clustering) and classifying them prior to processing enables the data to be divided into clusters that are required and not required for the target data processing, thereby reducing the volume of data to be processed.
In this research, we aim to develop a high-speed data clustering method, driven by statistical mechanical theory and machine learning theory methods.
Data clustering is an extremely essential process, and thus it has diverse application beyond data volume reduction, which is the objective of this research (for example, it can be used to extract communities in social networks, among other applications).
The proposed method is therefore expected to be useful for other research teams as well.
- Highly Efficient Computation Algorithm Design Theory based on a Combination of Computational Theory and Statistical Approximation Computational Theory
Designing a stochastic prediction system that can efficiently use big data is the ultimate goal of this research.
Such systems involve many problems in their internal computation with regard to optimizing combinations and graph theory that, although basic, are extremely challenging in terms of the amount of computations required; the overall processing could therefore suffer a serious bottleneck if this aspect of the computation is not made more efficient.
In this research, we aim to combine efficient computation algorithms based on computational theory, such as maximum flow computation, with statistical mechanical approximation theory to develop a new type of high quality computation algorithm specialized in big data analysis.